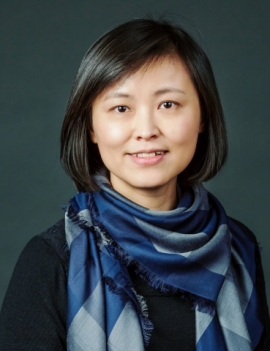
Mingyao Li, PhD
Dr. Li joined the biostatistics faculty in 2006 after receiving her PhD in Biostatistics from the University of Michigan in 2005. She is also a faculty member of the Genomics and Computational Biology (GCB) graduate program, and holds a secondary appointment in the Department of Statistics at Wharton. Her main research areas are statistical genetics and genomics, bioinformatics, and computational biology. The central theme of her current research is to use statistical and computational approaches to understand cellular heterogeneity in human-disease-relevant tissues, to characterize gene expression diversity across cell types, to study the patterns of cell state transition and crosstalk of various cells using data generated from single-cell transcriptomics studies, and to translate these findings to the clinics. In addition to methods development, Dr. Li is also interested in collaborating with researchers seeking to identify complex disease susceptibility genes and activing cell types. Her collaborative research includes age-related macular degeneration, Alzheimer's disease, cardiometabolic and heart disease, chronic kidney disease, melanoma, testicular cancer, and gene therapy for rare diseases. She leads the Laboratory for Statistical and Translational Genomics.
She is Director of Biostatistics for the Gene Therapy Program at Penn and advises biostatistics and bioinformatics analysis for various gene therapy studies. She is also Chair of the Graduate Program in Biostatistics. Dr. Li actively serves in the scientific community. She is Associate Editor of Annals of Applied Statistics, Statistics in Biosciences, PLOS Genetics, and PLOS Computational Biology, and was a regular member of the NIH Genomics, Computational Biology and Technology study section (2011-2017) and a member of the review committee of its Center for Inherited Disease Research (2013-2017).
Content Area Specialties
- Age-related macular degeneration
- Alzheimer's disease and related degeneration
- Cardiometabolic and heart disease
- Chronic kidney disease
- Cancer
- Gene therapy for rare diseases
Methods Specialties
- Statistical genetics and gene mapping of complex diseases
- Genome-wide association and next-generation sequencing
- Statistical methods for RNA sequencing
- Statistical methods for single-cell and spatial transcriptomics
- Machine learning methods for digital pathology
- Applications of machine learning