Hospitalizations Down 65 Percent, Patient Satisfaction Doubled: The Value of Community Health Workers
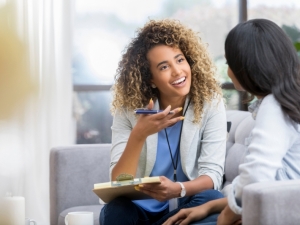
The term “social determinants of health” has become a part of our lexicon in healthcare; factors such as trauma, food insecurity and housing instability are widely known to influence high rates of chronic disease among disadvantaged people in the U.S. and elsewhere. But how can we best help patients deal with those factors, and how much we can improve their health by doing so? A Penn study of chronically ill, low-income patients and U.S. veterans has shown that community health workers — laypeople from local communities, who have experienced social issues and can aid high-risk patients in addressing them— can help reduce hospital stays by 65 percent and can double the rate of patient satisfaction with primary care.
The study is the first multi-center randomized controlled trial to demonstrate these kinds of improvements as results of a health-system-based social intervention, prompting the Wall Street Journal to comment that it offers some of the most compelling evidence to date that comprehensive community health worker programs can be effective in the U.S. “Improving health quality while lowering costly hospitalizations is what so many healthcare leaders are trying to achieve,” commented lead author Shreya Kangovi, MD, MS. “It’s remarkable that these results were achieved not by an expensive drug or technology, but from good old-fashioned social support.”
While community health workers are a rapidly growing healthcare workforce, many related programs struggle because they are not sufficiently standardized, they lack rigorous scientific evidence, or they only apply to one disease. With these issues in mind, the Penn team developed IMPaCT (Individualized Management for Patient-Centered Targets), a community health worker (CHW) model, to be highly standardized and scalable. In the study, community health workers delivered care at a VA medical center, a federally qualified health center, and an academic family practice clinic. Nearly 600 patients were randomly assigned to receive either standard primary care alone or six months of support from an IMPaCT worker plus standard primary care. Participants were residents of high-poverty zip codes in Philadelphia and had received a diagnosis of two or more targeted chronic diseases (diabetes, obesity, tobacco dependence, and hypertension), at least one of which was in poor control.
They received care in three stages: goal-setting, six months of tailored support, and connection with long-term support. Aiming for a nuanced approach, CHWs initiated goal-setting with open-ended interview questions such as, “Some people need a plan for getting enough food … how is that for you?” and “What do you think you’ll need in order to reach the health goal you set with your doctor?” Patients’ individualized goals became the basis for tailored action plans.
The results were striking. Compared to patients in the typical primary care group, those who received community health worker support were nearly twice as likely to report high-quality primary care, and spent fewer total days in the hospital at six months (155 days vs. 345 days) and at nine months (300 days vs. 471 days). Patients receiving community health worker support also had lower rates of repeat hospitalizations, including 30-day readmissions.
These findings are consistent with previous single-center randomized clinical trials measuring the effectiveness of the IMPaCT model, which showed notable results such as improved mental health, better access to quality care, reduced hospital readmissions and improvements in chronic-disease control. The IMPaCT model also recently received “gold status” in the Department of Veterans Affairs Shark Tank competition and will be replicated in VA medical centers where veterans face social challenges.
The study also brought other, unexpected benefits, adds Nandita Mitra, PhD, lead statistician and co-investigator. “Not only did these results truly impact people’s health. In addition, the study’s individualized goals posed interesting challenges that led us to develop new statistical methodology,” she remarked. During the course of the work, Dr. Mitra and then Biostatistics PhD student Edward Kennedy (now Assistant Professor of Statistics at Carnegie Mellon) developed causal inference methodology to specifically explore the effects of treatments on multiple outcomes simultaneously. Such designs can be particularly useful in patient-centered research, where different outcomes might be more or less important to different patients. The two proposed scaled effect measures that translate effects on multiple outcomes to a common scale. In an additional paper co-authored with Dr. Kangovi, they presented methods for estimating these scaled effects and for showing how treatment affects different outcomes in different ways. They also discussed methods for exploring how treatment effects may depend on additional factors. Their methods can benefit both randomized trials and observational studies.
Read the study in the Journal of the American Medical Association.
Read the statistical methods paper in Statistics in Medicine.